Qwen has been silently adding one model after the other. Each of its models comes packed with features so big and sizes so quantized that they are just impossible to ignore. After QvQ, Qwen2.5-VL, and Qwen2.5-Omni this year, the Qwen team has now released their latest family of models – Qwen3. This time they have released not one but EIGHT different models – ranging from a 0.6 billion parameter model to a 235 billion parameter model – competing with top models like OpenAI’s o1, Gemini 2.5 Pro, DeepSeek R1, and more. In this blog, we will explore the Qwen3 models in detail, and understand their features, architecture, training process, performance, and applications. Let’s get started.
What is Qwen3?
Developed by the Alibaba group, Qwen3 is the third generation of Qwen models that are designed to excel at various tasks like coding, reasoning, and language processing. The Qwen3 family consists of 8 different models consisting of 235 B, 30B, 32 B, 14 B, 8B, 4B, 1.7 B, and 0.6 B parameters. All the models are multi-modal meaning that they can take text, audio, image, and even video inputs and have been made freely available.
These models compete with top-tier models like o1, o3-mini, Grok 3, Gemini 2.5 Pro, and more. In fact this latest series of Qwen models not only outperforms the popular models but also marks a significant improvement over existing Qwen series models in comparable parameter categories. For example, the Qwen-30B-A3B (30 billion parameters with 3 billion activated parameters) model outperforms the QwQ-32B parameter model which has all its 32 billion parameters activated.
Introduction to the Qwen3 Models
The Qwen3 series comes packed with 8 models, out of which two are Mixture-of-Expert (MoE) models while the other 6 are dense models. The following table consists of details regarding all these models:
Model Name | Total Parameters | Activated Parameters (for MoE models) | Model Type |
Qwen3-235B-A22B | 235 Billion | 22 Billion | MoE (Mixture of Experts) |
Qwen3-30B-A3B | 30 Billion | 3 Billion | MoE (Mixture of Experts) |
Qwen3-32B | 32 Billion | N/A | Dense |
Qwen3-14B | 14 Billion | N/A | Dense |
Qwen3-8B | 8 Billion | N/A | Dense |
Qwen3-4B | 4 Billion | N/A | Dense |
Qwen3-1.7B | 1.7 Billion | N/A | Dense |
Qwen3-0.6B | 0.6 Billion | N/A | Dense |
In MoE models like Qwen3-235B-A22B and Qwen3-30B-A3B different parts of the network or “experts” get activated based on various inputs, making them highly efficient. In dense models like Qwen3-14B, all network parts are activated for every input.
Key Features of Qwen3
Here are some key highlights about the Qwen3 models:
1. Hybrid Approach
(i) Thinking Mode: This mode is useful when dealing with complex tasks involving multi-step reasoning, logical deduction, or advanced problem-solving. In this mode, the Qwen3 model breaks down the given problem into small, manageable steps to arrive at an answer.
(ii) Non-thinking Mode: This mode is ideal for tasks that demand quick and efficient responses like real-time conversations, information retrieval, or simple Q&A. In this mode, the Qwen3 models quickly generate replies based on their existing knowledge or just a simple web search.
This hybrid approach is now becoming quite popular among all the top-performing LLMs as the approach allows better utilization of LLMs capabilities and allows judicious use of tokens.

2. Flexibile Thinking
The latest Qwen3 series models give the users to also control the “depth” of thinking. This is the first of its kind feature, where the user gets to choose when the level of “thinking” resources that they wish to use for a given problem. This allows also users to better manage their budgets for a given task helping them to achieve an optimal balance between cost and quality.
3. MCP & Agentic Support
he Qwen3 models have been optimized for coding and agentic capabilities. These also come with enhanced support for Model Context Protocol (MCP). The Qwen3 models do so by showing better interaction capabilities with the external environment. They also come packed with improved ”tool calling” ability making them essential for building intelligent agents. In fact they have released “Qwen-Agent” a separate tool to allow the creation of intelligent agents using Qwen models.
4. Enhanced Pre and Post-Training
(i) Pre-training: Its pretraining process was a 3-step process. The first step involved training over 30 trillion tokens with a 4K context length. The second step involved training in STEM, coding, and reasoning tasks while the final step involved training with long-context data to extend context length to 32K tokens.
(ii) Post Training: The Qwen3 models that support the hybrid “thinking” approach support the 4-step reasoning process. The 4 steps involved a long chain-of-thought (CoT) cold start, reasoning-based reinforcement learning (RL), thinking mode fusion, and finally general reinforcement learning. The training of lightweight models involved distillation of the base models.

5. Accessibility Features
(i) Open Weight: All Qwen3 models are open weight under the Apache 2.0 license. This means that users are allowed to download, use, and even modify these models without any major restrictions.
(ii) Multi-lingual Support: The model currently supports over 119 languages and dialects, making it one of the few latest LLMs to focus on language inclusivity.
Qwen3 Models: Hands-on Applications
Now that we have discussed all their features in detail, it is time to explore the capabilities of Qwen3 models. We will test the following three models: Qwen3-235B-A22B, Qwen3-30B-A3B, and Qwen3-32B on the following three tasks:
- Complex logical reasoning
- Coding
- Image analysis
Let’s start.
Task 1: Complex Logical Reasoning
Prompt: “An astronaut travels from Earth to a distant star 8 light-years away at 0.8c (80% the speed of light), as measured from Earth’s frame. At the midpoint of the journey, the astronaut detours near a black hole, where strong gravitational time dilation occurs. The detour lasts 1 year in the astronaut’s frame, but in that region, time passes 10× slower compared to outside due to gravitational effects.
The astronaut claims that, including the detour, only 6 years passed for them during the entire journey.
Using special relativity and gravitational time dilation principles, evaluate whether the astronaut’s claim of “only 6 years passed” is consistent with the known relativistic effects. Provide a step-by-step explanation considering time experienced in both uniform motion and near the black hole.”
Model: Qwen3-30B-A3B
Output:
Review:
It’s impressive how fast this model works! It solves the problem step by step and explains each step simply. The model then gives detailed calculations relevant to the problem statement and then conclusively generates the result. It further explains the result and ensures that all points are covered effectively.
Task 2: Coding
Prompt: “Create a web page that helps users suggest the best outfit for them based on the weather, occasion, time of the day, and the price range.”
Model: Qwen3-235B-A22B
Output:

Review:
The model quickly generated the code for the web page with all the relevant inputs and it was easy to test the code by using the “artifacts” feature within the QwenChat interface. After the code was implemented, I just added the details to the generated webpage and got the outfit recommendations based on my requirements – all within a few seconds! This model showcased speed with accuracy.
Task 3: Image Analysis
Prompt: “Analyse the following images and arrange the models in the descending order of their performance on the “LiveCodeBench” benchmark.”
Model: Qwen3-32B
Output:
Review:
The model is great at image analysis. It scans the two images quickly and then based on it, the model delivers the result in the format that we requested it. The best part about this model is how quickly it processes the entire information and generates the output.
Qwen3: Benchmark Performance
In the last section, we saw the performance of 3 different Qwen3 models on 3 different tasks. All three models performed well and surprised me with their approach to problem-solving. Now let’s look at the benchmark performance of the Qwen models compared to the other top models and the previous models in the Qwen series.
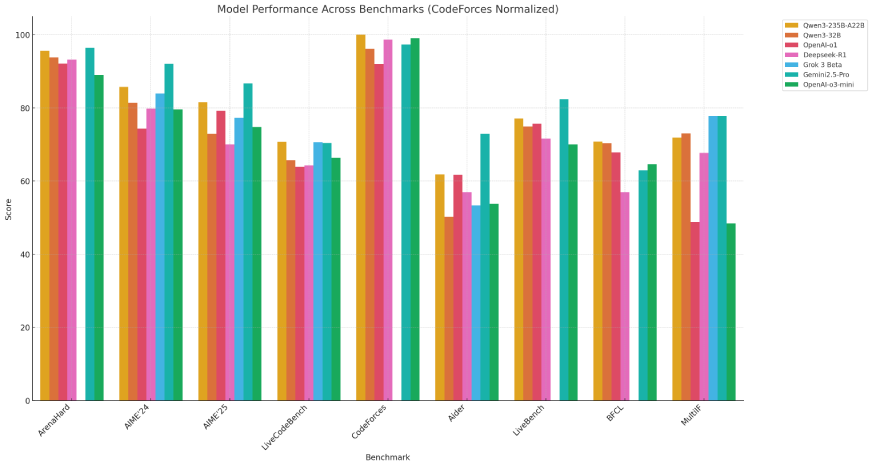
When compared to the top tier models like OpenAI-o1, DeepSeek-R1, Grok 3, Gemini 2.5 Pro – Qwen-235B-A22B stands as a clear champion, and rightfully so. It delivers stellar performance across coding and multilingual language support benchmarks.
In fact compact model Qwen3-32B too was able to outperform several models, making it a cost effective choice for many tasks.
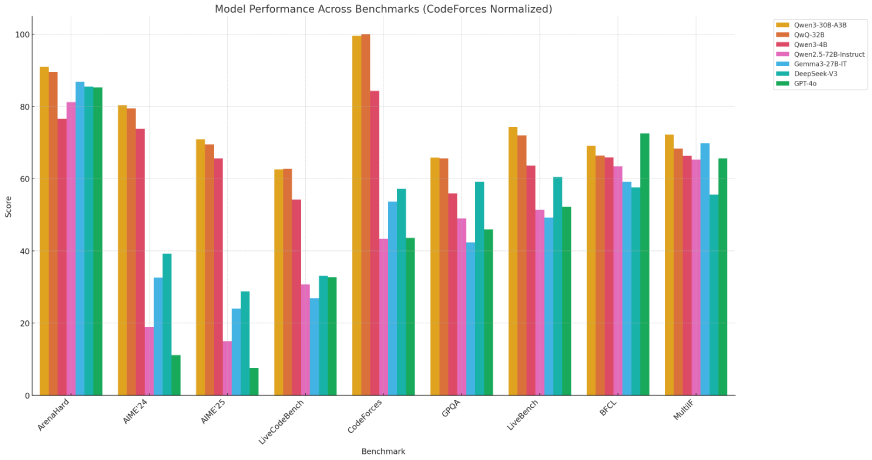
When compared with its predecessors, Qwen3 models: Qwen3-30B-A3B and Qwen3-4B outperform most of the existing models. These models do not only offer better performance but with their cost-efficient pricing, Qwen3 models truly are a step up over its previous versions.
Also Read: Kimi k1.5 vs DeepSeek R1: Battle of the Best Chinese LLMs
How to Access Qwen3 Models?
To access the Qwen3 models, you can use any of the following methods:
- Open QwenChat
Head to QwenChat.
- Select the Model
Select the model that you wish to work with from the drop-down present on the left side, in the middle of the screen.
- Accessing Post-trained & Pre-trained Models
To access the post-trained models and their pre-trained counterparts, head to Hugging Face, Modelscope, and Kaggle.
- Deploying the Models
For deployment, you can use frameworks like SGLang and vLLM.
- Accessing the Models Locally
To access these models locally, use tools like Ollama, LMStudio, MLX, llama.cpp, and KTransformers.
Applications of Qwen3 models
Qwen3 models are impressive and can be a great help in tasks like:
- Agent building: The Qwen3 models have been developed with enhanced function-calling features that would make them an ideal choice for developing AI Agents. These agents can then help us with various tasks involving finance, healthcare, HR, and more.
- Multilingual tasks: The Qwen3 models have been trained in various languages and can be a great value addition for developing tools that require support across multiple languages. These can involve tasks like real-time language translation, language analysis, and processing.
- Mobile applications: The small-sized Qwen3 models are significantly better than the other SLMs in the same category. These can be used to develop mobile applications with LLM support.
- Decision support for complex problems: The models come with a thinking mode that can help to break down complex problems like projections, asset planning, and resource management.
Conclusion
In a world where each latest LLM by top companies like OpenAI and Google has been about adding parameters, Qwen3 models bring efficiency even to the smallest of their models. These are free to try for everyone and have been made publicly available to help developers create amazing applications.
Are these models ground-breaking? Maybe not, but are these better? Definitely yes! Moreover, with flexible thinking, these models allow users to allocate resources according to the complexity of the tasks. I always look forward to Qwen model releases, because what they do is pack quality and features and punch out a result that most top models still haven’t been able to achieve.
Login to continue reading and enjoy expert-curated content.